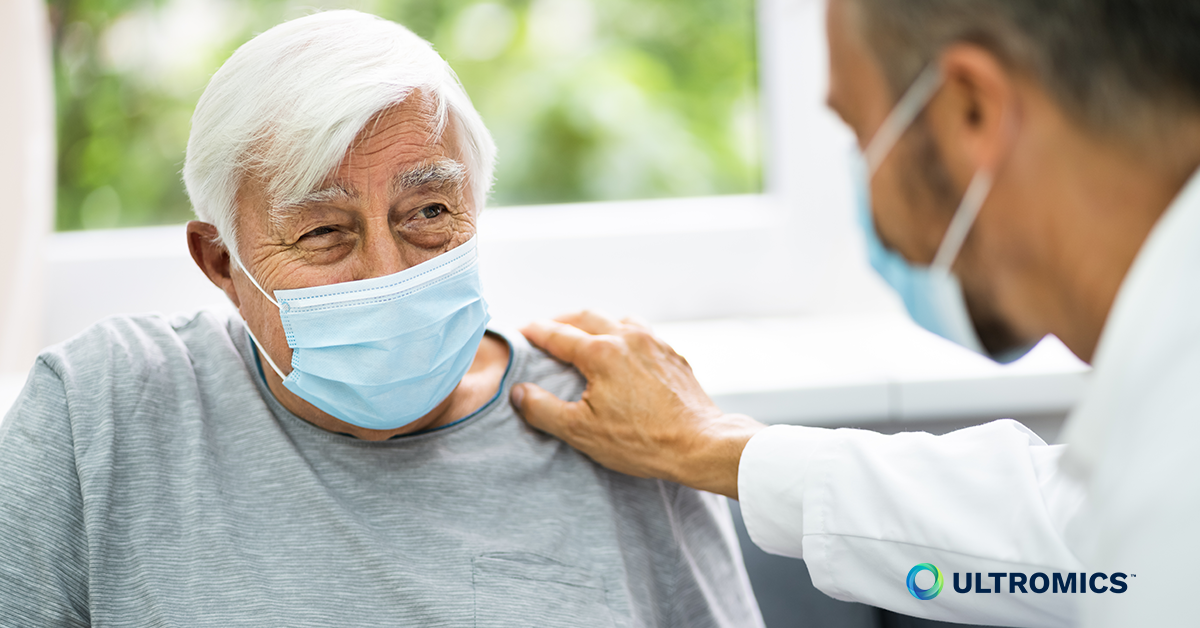
Global longitudinal strain and how AI can aid fight against heart failure
- | By Ultromics
- Strain
Heart failure (HF) is a prevalent yet silent epidemic, affecting 26 million people and costing global healthcare systems an estimated $65 billion every year1. While heart attacks are often sudden and can be symptomatic, heart failure is often considered a silent killer as it can build silently over time and treatment is often delayed to when the systolic burden has been compromised beyond reprieve.
Global longitudinal strain (GLS) echocardiography measurements are increasingly recognized as a more effective technique than conventional ejection fraction (EF) in detecting changes in left ventricular (LV) systolic function and predicting heart failure outcomes, however introducing GLS cardiac ultrasound into routine clinical practice has been fraught with challenges – including inter-operator variability, time and resource constraints.
Artificial intelligence (AI) can help overcome these challenges and move the benefits of GLS analysis from the pages of the journals to the front line in the fight against HF.
Heart Failure Epidemic
The global public health burden of HF is not only significant, but also continuously increasing. In the United States, around 5.7million people have HF, a figure that is expected to rise to 8 million by 20302. It is a trend being repeated around the world, particularly in developed countries.
It has a huge impact. In Europe, the Rotterdam Study showed a death rate of 11% at one year and 41% at year five4. In the US, the impact is even greater, the Framingham Heart Study found mortality rates of 20-30% at one year and 45-60% at five years3. These statistics have not changed in decades.
In 2012, HF was responsible for an estimated health expenditure of around $31 billion, more than 10% of the US’ total cardiovascular disease budget. As prevalence increases, so will the costs – as much as $69 billion by 20305
However, as cardiology communities the world over know, overcoming the challenge of HF is not easy, not least because of the lack of high-quality markers and predictors6.
Traditionally, teams have used EF to measure left ventricular (LV) systolic function which only represents a measure of systolic heart failure. LVEF is far from perfect and far from predictive of events. Changes in LVEF lag far behind effectively managed disease progression. This is evident in the growing number of patients with heart failure with preserved ejection fraction (HFpEF), now accounting for more than half of all patients with clinically diagnosed HF.
The need for more advanced, accurate measures and markers has never been more apparent. AI may be able to help by greatly simplifying and automating GLS measurements.
The Evidence for Global Longitudinal Strain and the Evaluation of Heart Failure
There is now an irrefutable and growing body of evidence to suggest that GLS is a significantly more useful HF metric than LVEF.
A paper published in the European Journal of Heart Failure in 2017 concluded that impaired GLS was indicative of diastolic dysfunction and elevated left ventricular filling pressures which is common in people with HFpEF7.
The authors, who also reported LV GLS was associated with biomarkers of wall stress, collagen synthesis, and diastolic dysfunction, said the findings indicated the presence of systolic and diastolic dysfunction even when LVEF was normal.
Later that year, a team from Duke University Medical Center reported a high prevalence of patients hospitalized with acute HFpEF had abnormal LV GLS. In addition, they discovered that abnormal LV GLS was associated with worse clinical outcomes at 30 days, though it is worth noting that this was no longer statistically significant at one year8.
“LV GLS may be a useful tool for identifying a cohort of HFpEF patients with more overt myocardial dysfunction who are at risk for worse outcomes following a hospitalization for HF,” concluded the authors of the Duke University Medical Center study.
This role of GLS in predicting disease severity was further highlighted in a study of almost 2,200 patients, published last month9.
The observational, prospective cohort study looked at the GLS, as measured during an echo, of 2,186 people with stages A to D HF according to the American Heart Association (AHA) criteria8. The team found that GLS was not only associated with HF severity, but during a median follow up period of just over three years, was predictive of all-cause mortality and cardiac death8.
A 2019 review of the evidence concluded that GLS had “greater prognostic value” than LVEF.[8] “GLS adds incremental value to EF in the prediction of adverse outcomes,” the study authors wrote.
“Comprehensive evaluation of GLS in HF patients provides additive prognostic information and can play a significant role in improving risk stratification in chronic systolic heart failure.”
But while they said they were “hopeful” that GLS would become the standard measurement for HF in the future, they also acknowledged the challenges of bringing it into routine practice.
Advancing Clinical Practice With Global Longitudinal Strain With the Help of AI
However, effectively utilizing global longitudinal strain for the measurement of HF is not without its challenges. Most strain software still requires at least some level of manual approval and contouring, leaving calculations vulnerable to human error, and there exists differences in GLS measurements across the different ultrasound equipment vendors.
Echocardiographers are also faced with time constraints. The average echo exam can take approximately 20-30 minutes of imaging time, so the addition of (strain) measurements to the standard protocol along with the time taken to acquire them — particularly as teams attempt to clear COVID-related backlogs — can be problematic.
Cost is another major barrier to the wide-scale adoption of global longitudinal strain analysis and reporting. Most vendors offer strain modules for their ultrasound machines, but these can be prohibitively expensive when considering the number of devices and software licenses required in relation to the practical application to patient populations. Additionally, those that have started to perform strain have discovered the cost burden of decreased productivity when staff perform strain routinely on all exams. But AI can help on many fronts.
AI can automate the parts of the process that lead to inter-observer variability. Combining the latest knowledge of GLS with the innovation of cloud-based AI technology may be the key to improving outcomes and reducing the costs associated with the silent challenging epidemic of HF.
AI services like EchoGo can automate echocardiography analysis, streamline workflow and increase throughput, enabling echocardiographers to better measure GLS more routinely and ubiquitously without impacting productivity.
Over the last few years, researchers have demonstrated the value of GLS in measuring disease severity and predicting outcomes. Patients can benefit from this discovery if the technology is seamlessly introduced into clinical practice.
There are always challenges to implementation, but there are also solutions. Combining the latest knowledge of GLS with the innovation of cloud-based AI technology is the key to improving outcomes and reducing the costs associated with the silent challenging epidemic of HF.
References:
- Savarese G, and Lund LH. Global Public Health Burden of Heart Failure. Cardiac Failure Review. 2017;3(1):7-11.
- Savarese G, and Lund LH. Global Public Health Burden of Heart Failure. Cardiac Failure Review. 2017;3(1):7-11.
- Bytyçi I, Bajraktari G. Mortality in heart failure patients. Anatolian Journal of Cardiology [Internet]. 2015 Jan 1;15(1):63–8.
- Hofman A, Breteler MMB, van Duijn CM, Krestin GP, Pols HA, Stricker BHCh, et al. The Rotterdam Study: objectives and design update. European Journal of Epidemiology. 2007;22(11):819–29.
- Savarese G, and Lund LH. Global Public Health Burden of Heart Failure. Cardiac Failure Review. 2017;3(1):7-11.
- DeVore AD, McNulty S, Alenezi F, et al. Impaired left ventricular global longitudinal strain in patients with heart failure with preserved ejection fraction: insights from the RELAX trial. European Journal of Heart Failure [Internet]. 201719(7):893–900.
- Buggey J, Alenezi F, Yoon HJ, et al. Left ventricular global longitudinal strain in patients with heart failure with preserved ejection fraction: outcomes following an acute heart failure hospitalization. ESC Heart Failure. 2017;4(4):432–9.
- Tröbs SO, Prochaska JH, Schwuchow-Thonke S, et al. Association of Global Longitudinal Strain With Clinical Status and Mortality in Patients With Chronic Heart Failure. JAMA Cardiology [Internet]. 2021;6:448–56.
- Ashish K, Faisaluddin M, Bandyopadhyay D, et al. Prognostic value of global longitudinal strain in heart failure subjects: A recent prototype. IJC Heart & Vasculature. 2019;22:48–9.
*This article was originally published on Diagnostic and Interventional Cardiology
Curious about upcoming research and innovation?
Sign up to hear about the latest news.

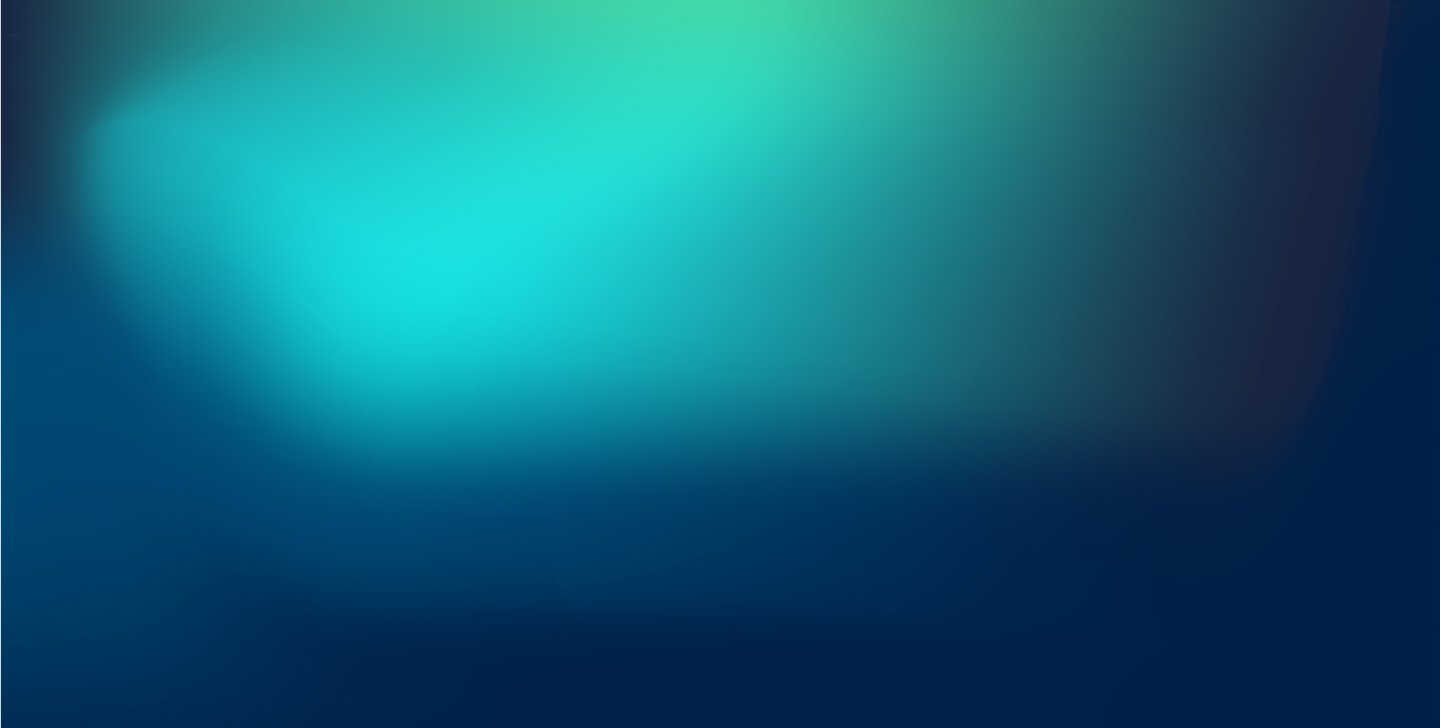