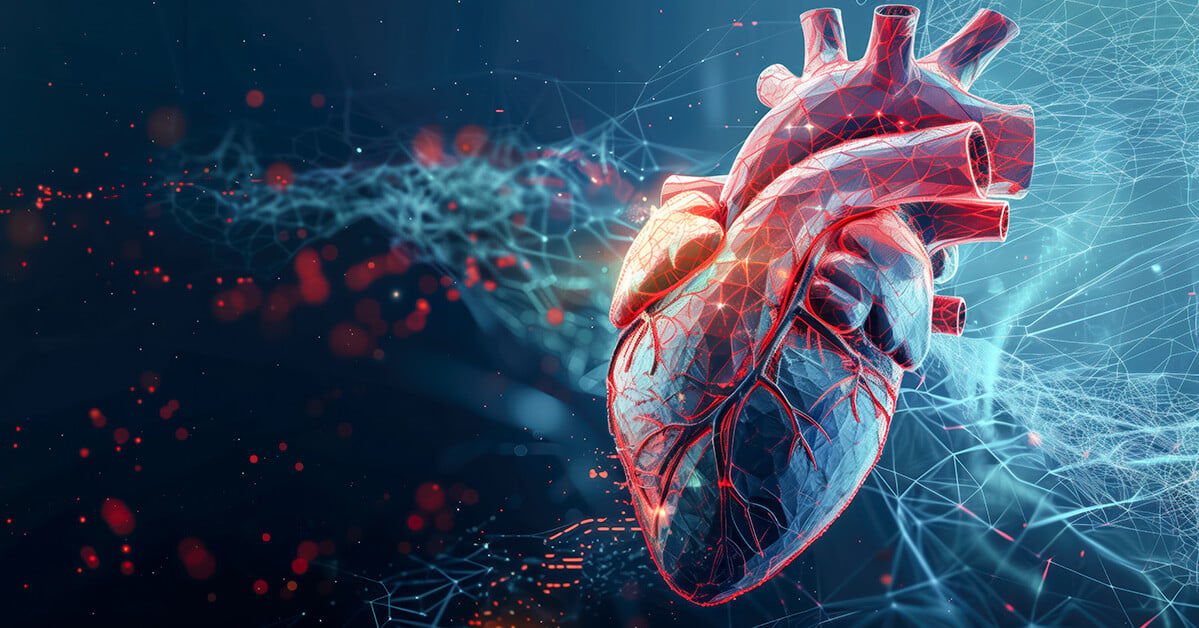
Study Confirms AI-Based Detection of HFpEF is Linked to Cardiac Outcomes
- | By Ultromics
- Press Release
Oxford, UK – Tuesday, 30th July, 2024 – A novel AI model capable of detecting heart failure with preserved ejection fraction (HFpEF) from a single echocardiographic video clip has been shown to improve the prediction of cardiac mortality and hospitalization, according to research published today in Journal of the American Society of Echocardiography (JASE).
HFpEF accounts for approximately 50% of heart failure diagnoses and frequently leads to hospitalization and increased mortality rates.1 Despite its prevalence, HFpEF is notoriously difficult to diagnose and manage due to the lack of specific symptoms and limitations of traditional methods of detection.
Using AI, cardiologists have achieved more accurate risk stratification and diagnostic classification in patients with HFpEF, enhancing early intervention for patients with this challenging subtype of heart failure.
The retrospective, multi-site study, conducted by the Mayo Clinic, followed 1,284 patients over a median period of 3.4 years. The study revealed that positive AI outputs were significantly associated with higher risks of heart failure hospitalization and cardiac mortality.2 Furthermore, the AI model allowed classification of risk in most of the cases in which clinical scores were nondiagnostic, improving predictive accuracy and enhancing patient management strategies.2
Key Findings
- High Sensitivity and Specificity: The AI model demonstrated impressive sensitivity (89.8%) and specificity (86.3%) in distinguishing HFpEF cases from controls, significantly outperforming traditional clinical scores.2
- Risk Stratification: Positive AI outputs were associated with a higher risk of heart failure hospitalization (HR 3.76) and cardiac mortality (HR 5.55), emphasizing the model's ability to predict adverse clinical outcomes.2
- Reclassification Capability: The AI model successfully reclassified 91% of nondiagnostic H2FPEF outputs and 92% of nondiagnostic HFA-PEFF outputs, showcasing its utility in refining risk assessment for HFpEF patients.2
Figure 1. Risk for HF hospitalization was higher in patients with positive and uncertain AI model output (top). Those with output scores that placed them in higher quartiles had incrementally greater risk for HF hospitalization (bottom), accounting for competing risk for death.
Figure 2. Risk for HF hospitalization was higher in patients with positive H2FPEF outputs (top), but many (n = 776) had nondiagnostic outputs. Application of the AI model was able to reclassify 708 (91%) of the nondiagnostic H2FPEF outputs (bottom).
Ross Upton, PhD, founder, CEO, and Chief Scientific Officer of Ultromics, emphasized the potential of AI in transforming HFpEF management: “This study highlights the significant advancements AI can bring to cardiovascular care, in particular, the association with HFpEF patient outcomes is a crucial finding. With the global shift toward population aging, heart conditions like HFpEF are becoming more prevalent and often overlooked, increasing the burden on hospitals. As a result, there is a critical need for advanced tools that can facilitate early detection and intervention.”
Patricia A. Pellikka, M.D., a cardiologist at Mayo Clinic and lead study author, added, “Clearly identifying heart failure with preserved ejection fraction and its level of severity has been a challenge for clinicians. Our study demonstrates that the AI model not only accurately identifies patients at increased risk of heart failure hospitalization and cardiac mortality but also provides valuable prognostic information that could enhance clinical decision-making. This technology holds promise for improving patient outcomes through early and precise detection of HFpEF."
The findings suggest that EchoGo® Heart Failure can significantly enhance the early detection and management of patients with HFpEF. By identifying those at higher risk of hospitalization and mortality, the AI provides clinicians with critical information to initiate timely and appropriate interventions. This capability is crucial for improving patient care and outcomes through timely and targeted management strategies.
As the first and only AI echocardiographic model to produce outputs discriminating HFpEF associated with clinical outcomes, these findings open new avenues for prospective studies and external validations. Future research will focus on confirming these retrospective results, validating the AI model’s outputs in diverse echocardiographic laboratories, and exploring the implications for patient management.
EchoGo® Heart Failure provides an easy-to-use mechanism for supporting earlier identification of this heterogeneous disease, expediting the movement of patients through the clinical pathway to appropriate diagnosis and treatment. It has been built, evaluated, and validated as part of a multi-site, international collaboration with a diverse source data population spanning 7 major clinical sites.3
The technology has been cleared for commercial use and awarded Breakthrough Device Designation by the FDA. Its clinical impact is further recognized by the assignment of a Category III Current Procedural Terminology (CPT) code (0932T) and New Technology Add-on Payment (NTAP) code (XXE2X19). Learn more about EchoGo® Heart Failure.
Mayo Clinic has a financial interest in the technology referenced in this press release. Mayo Clinic will use any revenue it receives to support its not-for-profit mission in patient care, education and research.
Read the full publication on JASE
References:
- Pfeffer MA et al. Circ Res. 2019;124(11):1598-617.
- Cassiani C, Huntley GD, Castrichini M, et al. J Am Soc Echocardiogr. 2024; DOI: 10.1016/j.echo.2024.06.016.
- Akerman, Upton, Pellikka et al. JACC Adv. 2023 Aug, 2 (6) 100452.

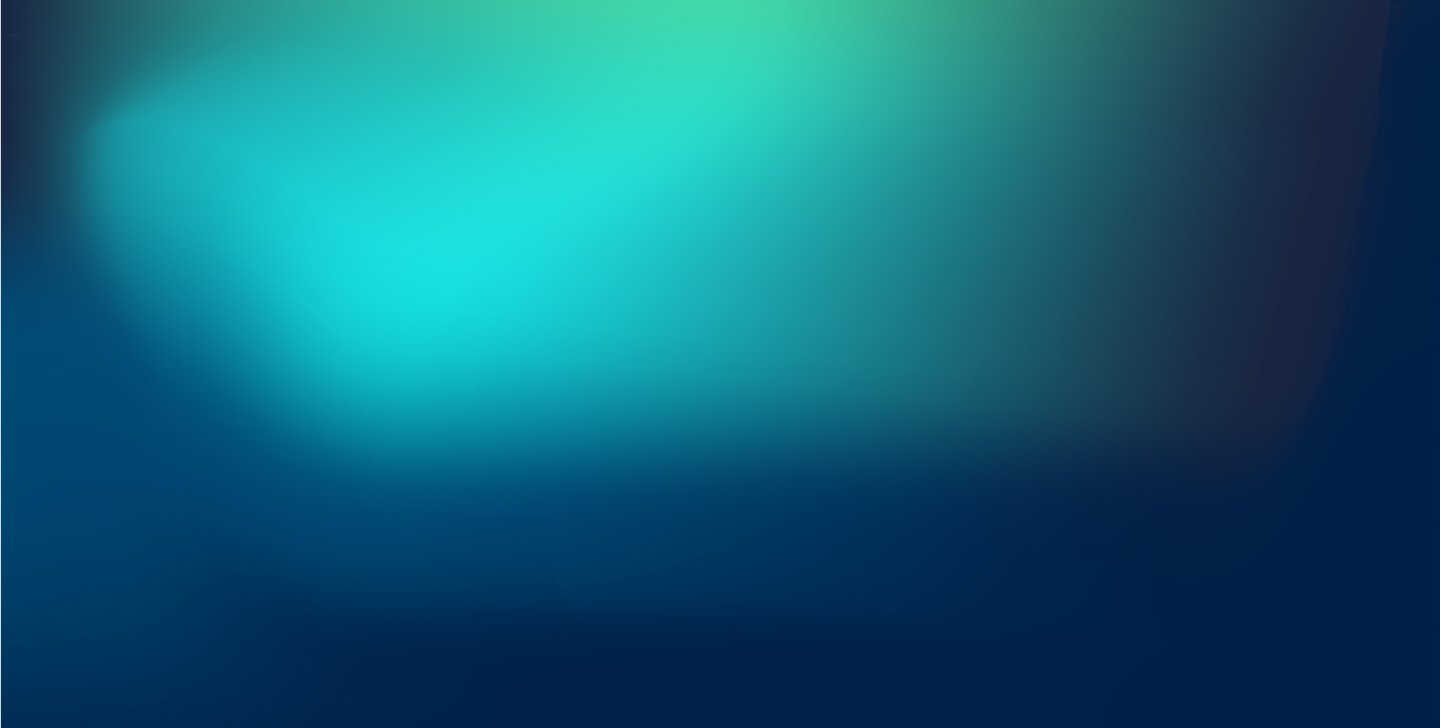