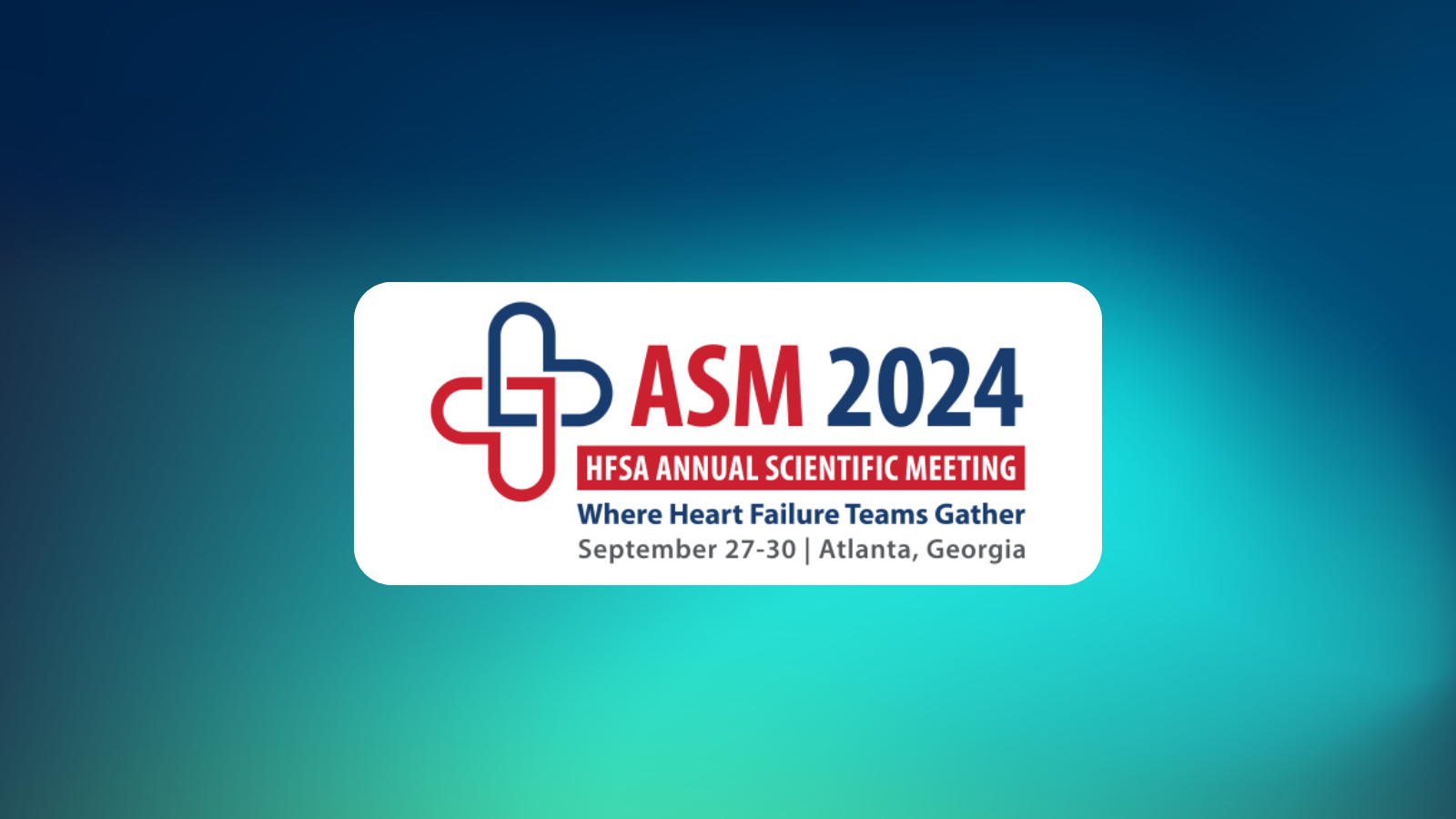
AI-based Echocardiographic Assessment Is Associated with HF Hospitalization and Cardiac Mortality in HFpEF
- | By Ultromics
C. Cassianni 1, G. D. Huntley 1, M. Castrichini 1, A. P. Akerman 2, M. Porumb 2, C. G. Scott 1, H.N. Davison 1, W. Hawkes 2, G. Woodward 2, B. Borlaug 1, R. Upton 2, P. A. Pellikka 1
1 Mayo Clinic, MN, United States of America; 2 Ultromics Ltd, United Kingdom
Objectives
Heart failure with preserved ejection fraction (HFpEF) is a clinical syndrome with increasing prevalence, poor 5-year survival rates, high re-admission rates, and substantial morbidity. Advances in development and implementation of artificial intelligence (AI) in the HFpEF diagnostic pathway show promise but require progressive development and validation to ensure clinical utility and meaningful impact. We therefore examined the association between version 2 of an FDA-approved HFpEF diagnostic aid with patient outcomes.
Methods
A three-dimensional convolutional neural network was developed using retrospective, multi-site, and multi-national cohort data (Mayo Clinic, USA, and NHS, UK) to automatically detect HFpEF (EchoGo Heart Failure; Ultromics Ltd 1). HFpEF cases represented patients with EF ≥50%, evidence of increased intra-cardiac filling pressure, and a diagnosis of heart failure (ICD-9/10) within one year of the echocardiogram. Controls represented patients with EF ≥50%, but no evidence of increased intra-cardiac filling pressure or diagnosis of HF. Version 2 of the AI model provided a continuous probability of HFpEF to support the existing binary classification for high and low likelihood of HFpEF, and an uncertain output as risk mitigation. Version 2 also implemented more rigorous augmentation of the model in pre-processing based on real-world experience of the model in clinical implementation. Model performance and association with outcomes was examined in a multi-site retrospective dataset1, consisting of 646 patients with HFpEF and 638 patients without HFpEF. Incident HF hospitalization was obtained from electronic health record chart review. Mortality was obtained from the National Death Index, and causes of cardiac deaths were manually reviewed. Cardiac mortality and HF hospitalization were plotted accounting for death as a competing risk, and Fine and Gray method was used to estimate the hazard ratios (HRs) adjusted for differences in age and sex between groups. Integration of AI model and existing clinical scores (H2FPEF2) are examined.
Results
Table 1 presents model performance between version 1 and version 2 in a previously described independent test population (Table 2). Version 2 of the model produced 95 uncertain outputs, compared to 94 in version 1 (both 7.4%). Discrimination, classification, and calibration were all improved in the 2nd version of the model. Among the 1,284 patients followed for a median of 3.4 years (interquartile range, 1.7-6.5 years), there were 252 HF hospitalizations and 540 deaths. Figure 1 demonstrates the risk for HF hospitalization according to AI model categorical output (top) and quartiles of continuous probability output (bottom). Positive AI output was associated with a higher risk for HF hospitalization than negative output (HR, 3.76; 95% CI, 2.71-5.21; P < .001) and likewise for uncertain output (HR, 2.79; 95% CI, 1.60-4.62; P < .001). Similarly, cardiac mortality (n=135) was higher in patients with positive output (HR, 5.55; 95% CI, 3.28-9.37; P < .001); patients with an uncertain output tended to have a higher mortality (HR, 2.22; 95% CI, 0.94-5.24; P = .07). Patients with higher continuous probability outputs demonstrated incrementally higher risk for cardiac mortality (fourth quartile vs first quartile: HR, 11.65; 95% CI, 4.65-29.20; P < .0001). Figure 2 demonstrates the application of the AI model to patients with nondiagnostic H2FPEF outputs for stratification of risk of HF hospitalization. This sequential approach allowed the classification of all but 68 of the 776 patients (8.8%) and demonstrated similar associations with patient outcomes.
Conclusion
The AI model was associated with higher risk of HF hospitalization and cardiac mortality, with incremental risk according to categorical and continuous probability outputs. Integration of the AI model into the diagnostic pathway permitted reclassification of previously indeterminate patient risk, and identification of patients at higher risk of hospitalization.