Kit M. Bransby, Arian Beqiri, Woo-Jin Cho Kim, Jorge Oliviera, Agisilaos Chartsias, Alberto Gomez
Read the full publication here.
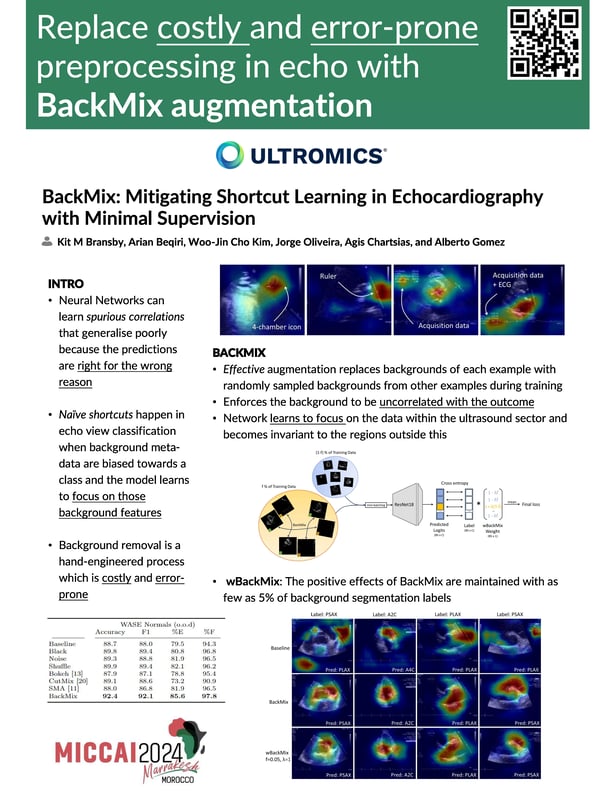
Background
Neural networks can learn spurious correlations that lead to
the correct prediction in a validation set, but generalise poorly because
the predictions are right for the wrong reason. This undesired learning of
naive shortcuts (Clever Hans effect) can happen for example in echocardiogram view classification when background cues (e.g. metadata) are
biased towards a class and the model learns to focus on those background features instead of on the image content. We propose a simple,
yet effective random background augmentation method called BackMix,
which samples random backgrounds from other examples in the training
set.
Method
By enforcing the background to be uncorrelated with the outcome,
the model learns to focus on the data within the ultrasound sector and
becomes invariant to the regions outside this. We extend our method in a
semi-supervised setting, finding that the positive effects of BackMix are
maintained with as few as 5% of segmentation labels. A loss weighting
mechanism, wBackMix, is also proposed to increase the contribution of
the augmented examples.
Results
We validate our method on both in-distribution
and out-of-distribution datasets, demonstrating significant improvements
in classification accuracy, region focus and generalisability. Our source
code is available at: https://github.com/kitbransby/BackMix
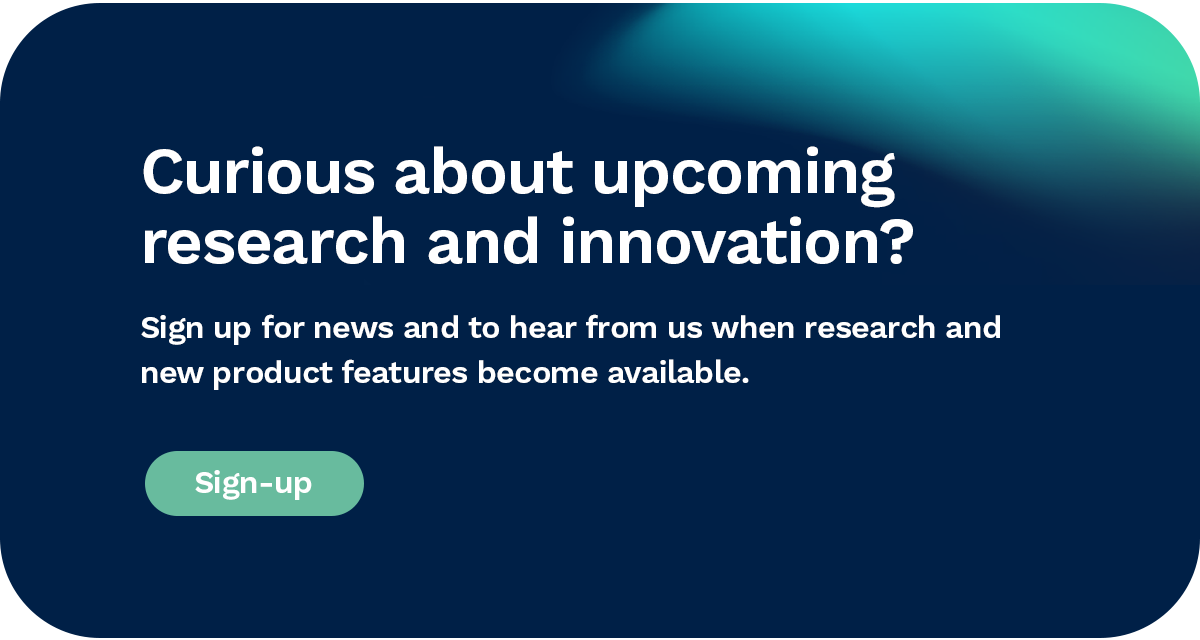