Katarina Yaros1, Matthew Segar2, Vinayak Subramanian1, Alvin Chandra1, Thomas Koshy1, Ross Upton3, Ashley Akerman3, Ambarish Pandey1
1 UT Southwestern Medical Center, 2 Texas Heart Institute, 3 Ultromics
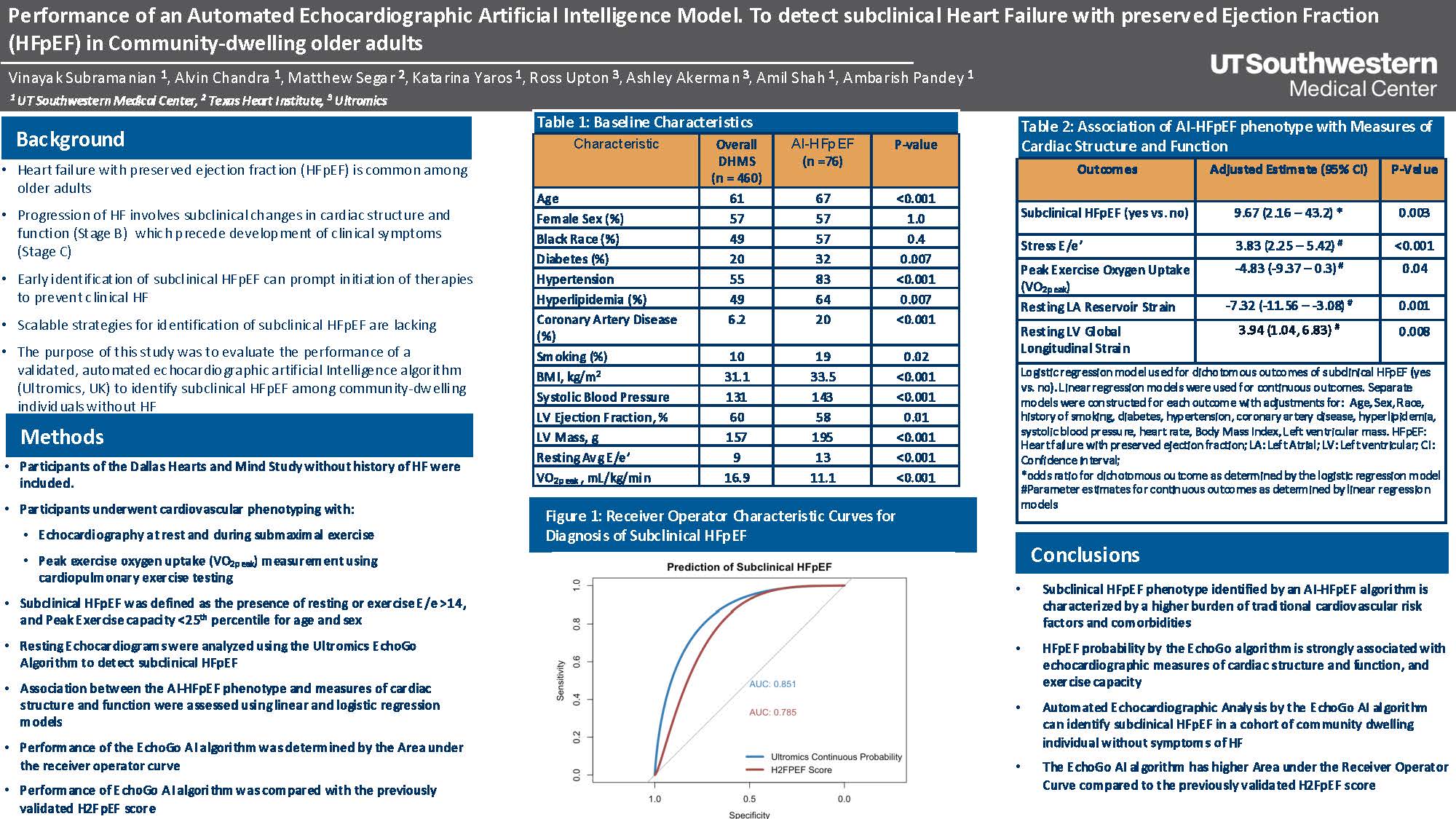
See full-size poster.
Background
• Heart failure with preserved ejection fraction (HFpEF) is common among
older adults.
• Progression of HF involves subclinical changes in cardiac structure and
function (Stage B) which precede development of clinical symptoms
(Stage C).
• Early identification of subclinical HFpEF can prompt initiation of therapies
to prevent clinical HF.
• Scalable strategies for identification of subclinical HFpEF are lacking.
• The purpose of this study was to evaluate the performance of a
validated, automated echocardiographic artificial Intelligence algorithm
(Ultromics, UK) to identify subclinical HFpEF among community-dwelling
individuals without HF.
Method
• Participants of the Dallas Hearts and Mind Study without history of HF were included.
• Participants underwent cardiovascular phenotyping with:
• Echocardiography at rest and during submaximal exercise.
• Peak exercise oxygen uptake (VO2 peak) measurement using cardiopulmonary exercise testing.
• Subclinical HFpEF was defined as the presence of resting or exercise E/e >14, and Peak Exercise capacity <25th percentile for age and sex.
• Resting Echocardiograms were analyzed using the Ultromics EchoGo Algorithm to detect subclinical HFpEF.
• Association between the AI-HFpEF phenotype and measures of cardiac structure and function were assessed using linear and logistic regression models.
• Performance of the EchoGo AI algorithm was determined by the Area under the receiver operator curve.
• Performance of EchoGo AI algorithm was compared with the previously validated H2FpEF score.
Results
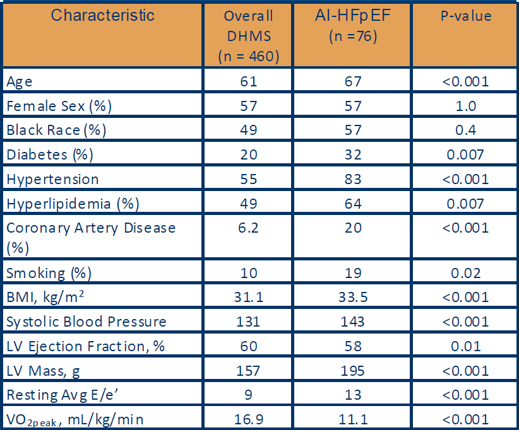
Table 1. Baseline Characteristics
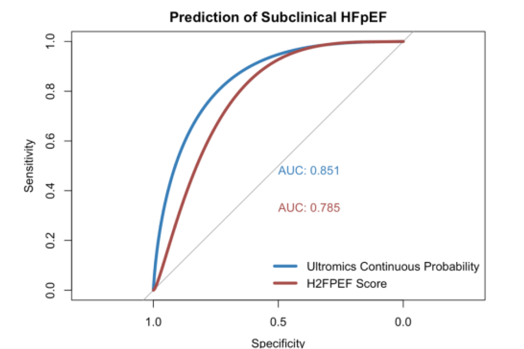
Figure 1. Receiver Operator Characteristic Curves for Diagnosis of Subclinical HFpEF
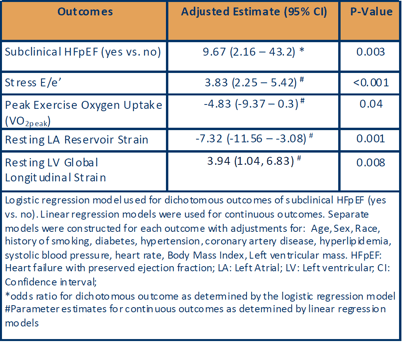
Table 2. Association of AI-HFpEF phenotype with Measures of Cardiac Structure and Function
Conclusions
• Subclinical HFpEF phenotype identified by an AI-HFpEF algorithm is characterized by a higher burden of traditional cardiovascular risk factors and comorbidities.
• HFpEF probability by the EchoGo algorithm is strongly associated with echocardiographic measures of cardiac structure and function, and exercise capacity.
• Automated Echocardiographic Analysis by the EchoGo AI algorithm can identify subclinical HFpEF in a cohort of community dwelling individual without symptoms of HF.
• The EchoGo AI algorithm has higher Area under the Receiver Operator Curve compared to the previously validated H2FpEF score.