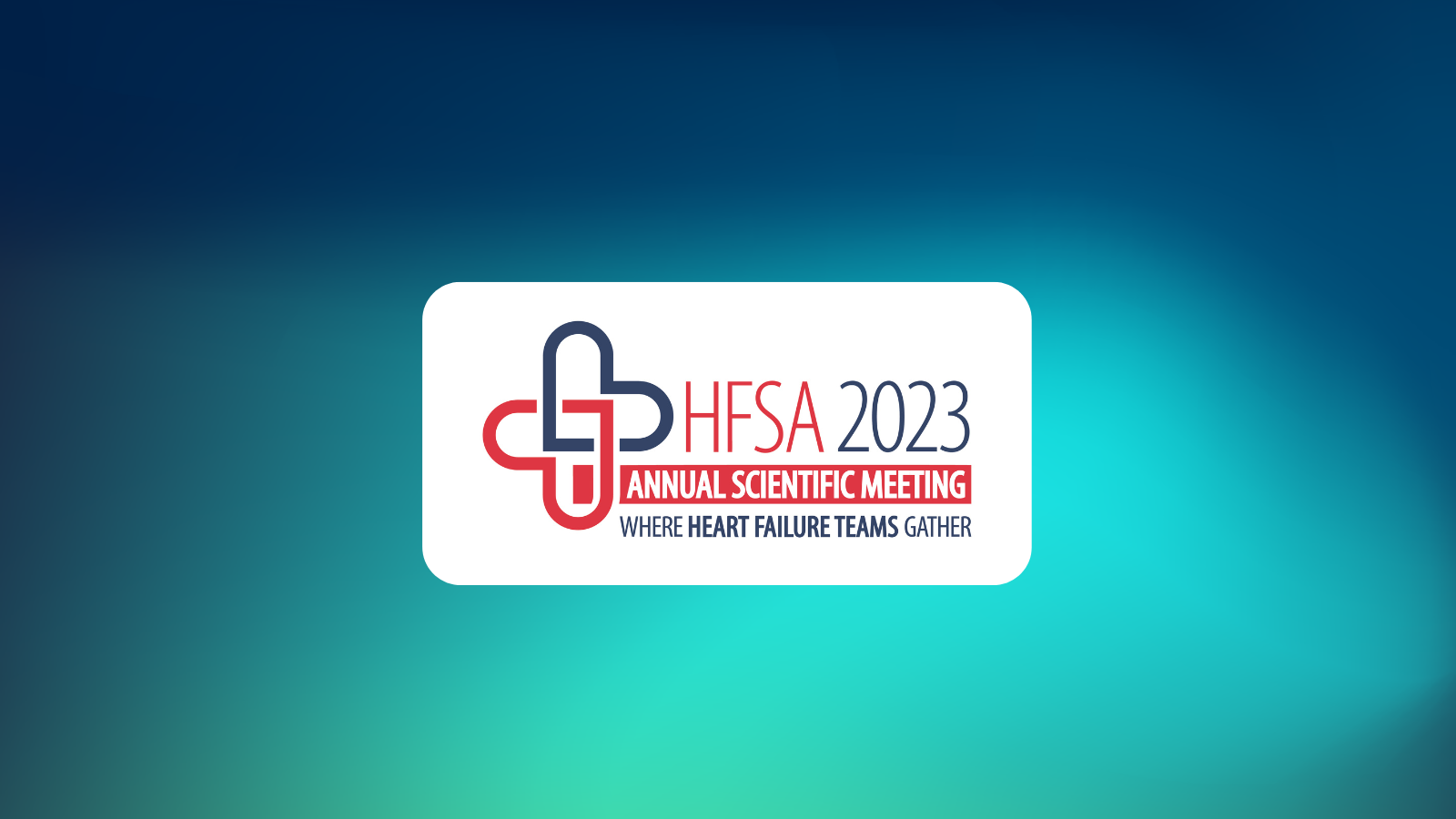
Clinical Utility Of An AI-Based Model For Detecting Heart Failure With Preserved Ejection Fraction (HFpEF) Through Decision Curve Analysis
- | By Ultromics
Akerman, A.P*., Porumb, M*., Scott, C.G#., Beqiri, A*., Chartsias, A*., Ryu, A.J#., Hawkes, W*., Huntley, G.D#., Arystan, A.Z#., Kane, G.C#., Pislaru, S.V#., Lopez-Jiminez, F#., Sarwar, R*,^., O’Driscoll, J*, $., Leeson, P*, ^., Upton, R*., Woodward, G*., Pellikka, P.A#.
*Ultromics Ltd, Oxford, UK, #Mayo Clinic, MN, USA, ^ University of Oxford, UK, $ Canterbury Christ Church University, UK
Purpose/Objectives
Heart failure with preserved ejection fraction (HFpEF) is a clinical syndrome with increasing prevalence, poor 5-year survival rates, high re-admission rates, and substantial morbidity. Echocardiography is critical in the HFpEF diagnostic pathway, but algorithms for echocardiographic interpretation, and the integration into broader clinical decision making are limited by discordant or incomplete data. This leads to variable diagnostic capacity, increasing requirements for further confirmatory testing or incorrect patient management.
A three-dimensional convolutional neural network was developed to automatically detect HFpEF using only the apical four-chamber videoclip (EchoGo Heart Failure; Ultromics Ltd). Model development utilized retrospective, multi-site, and multi-national cohort data (Mayo Clinic, USA; NHS, UK). Echocardiogram databases and electronic medical records were used to identify patients with preserved ejection fraction (≥50%), and evidence of increased intra-cardiac filling pressure, and a diagnosis of heart failure (ICD-9/10) within one year of the echocardiogram or lack thereof (cases and controls, respectively). In an independent testing dataset comprised of multi-site retrospective data from Mayo Clinic Health System (USA), the AI model was compared to clinically validated algorithms (HFA-PEFF Score and H2FPEF Score) with respect to classification performance (sensitivity and specificity) and the impact on clinical decision making (decision curve analysis).
Materials & Methods
A novel AI model to detect HFpEF provided more diagnostic outputs than clinically validated algorithms. Patients with indeterminate outputs by clinical algorithms were often correctly re-classified by the AI model. Use of such a model in a screening paradigm or to support uncertain diagnoses could facilitate correct patient management.
Results
Patient demographics for the 2971 cases and 3785 controls utilized for training and validation of the AI model, and 646 cases and 638 controls utilized for independent testing are presented in Table 1. The AI model demonstrated excellent discrimination performance in all datasets, with AUROC between 0.91 and 0.97, and very good sensitivity (mean: 87.8% [95% CI: 84.5, 90.9]) and specificity (81.9% [78.2, 85.6]) on 1190/1284 patients in the training dataset (uncertain in 7.3%). The HFA-PEFF and H2FPEF scores also demonstrated very good sensitivity (84.1% [78.1, 91.4] and 98.2% [96.3, 99.8]) and specificity (99.7% [98.8, 100] and 74.0% [66.9, 79.0]), but were indeterminate in 820 (63.9%) and 776 (60.4%) patients, respectively. When indeterminate patients according to the HFA-PEFF score or H2FPEF score were assessed by the AI model, 610 (74.4%) and 571 (73.6%) of patients were correctly reclassified, respectively.
Summary/Conclusion
In the testing dataset, modelling patient management decisions (e.g., prescription of SGLT2i) based on the combined diagnostic capacity of the AI HFpEF model and the HFA-PEFF or H2FPEF score, compared with the clinical score alone, resulted in more true positives being identified per 100 in the target population.