Hadrien Reynaud, Qingjie Meng, Mischa Dombrowski, Arijit Ghosh, Thomas Day, Alberto Gomez, Paul Leeson, Bernhard Kainz
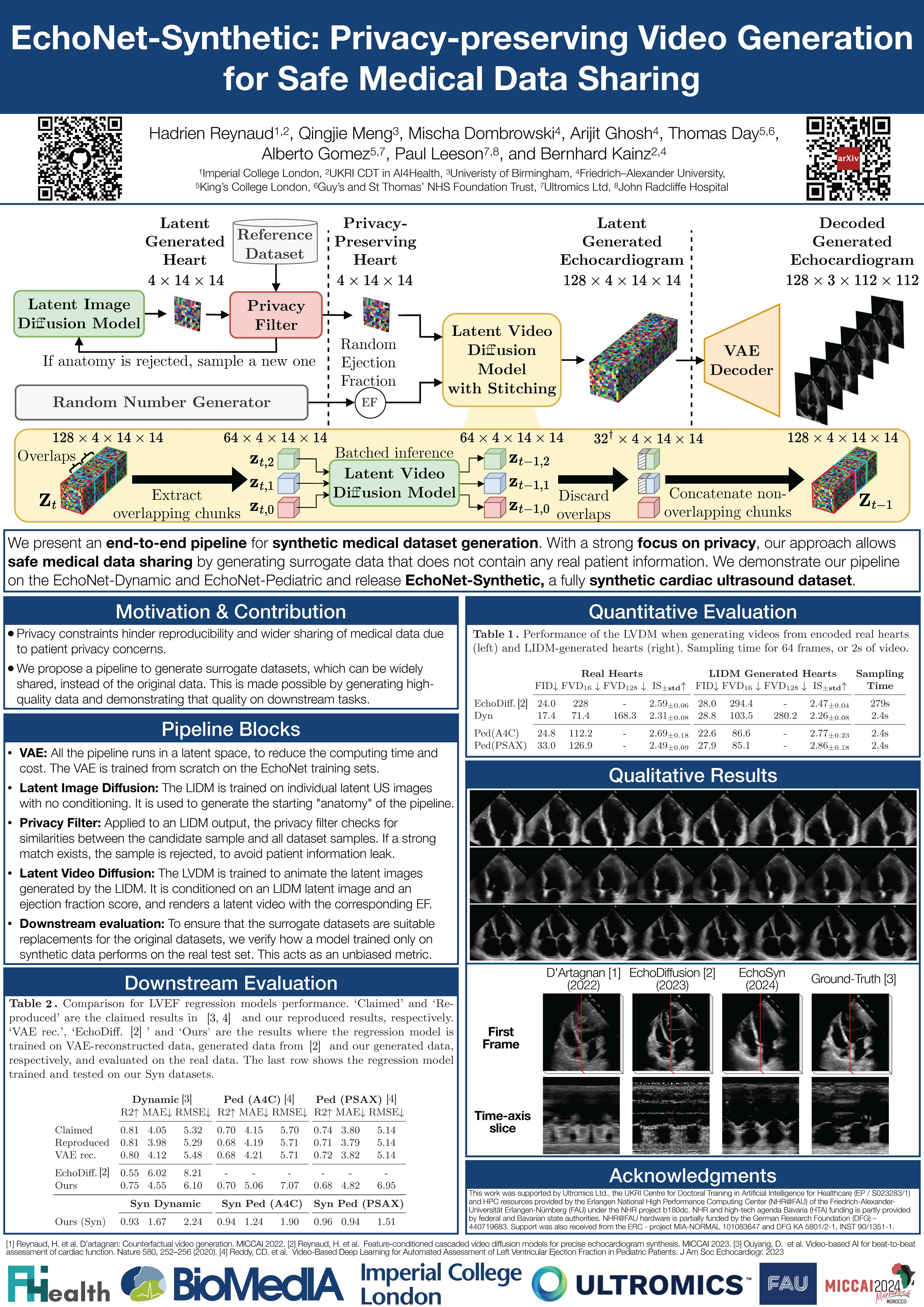
See full-size poster.
Background
To make medical datasets accessible without sharing sensitive patient information, we introduce a novel end-to-end approach for generative de-identification of dynamic medical imaging data. Until now, generative methods have faced constraints in terms of fidelity, spatio-temporal coherence, and the length of generation, failing to capture the complete details of dataset distributions. We present a model designed to produce high-fidelity, long and complete data samples with near-real-time efficiency and explore our approach on a challenging task: generating echocardiogram videos. We develop our generation method based on diffusion models and introduce a protocol for medical video dataset anonymization. As an exemplar, we present EchoNet-Synthetic, a fully synthetic, privacy-compliant echocardiogram dataset with paired
ejection fraction labels. As part of our de-identification protocol, we evaluate the quality of the generated dataset and propose to use clinical downstream tasks as a measurement on top of widely used but potentially biased image quality metrics. Experimental outcomes demonstrate that EchoNet-Synthetic achieves comparable dataset fidelity to the actual dataset, effectively supporting the ejection fraction regression task. Code, weights and dataset are available at https://github.com/
HReynaud/EchoNet-Synthetic.
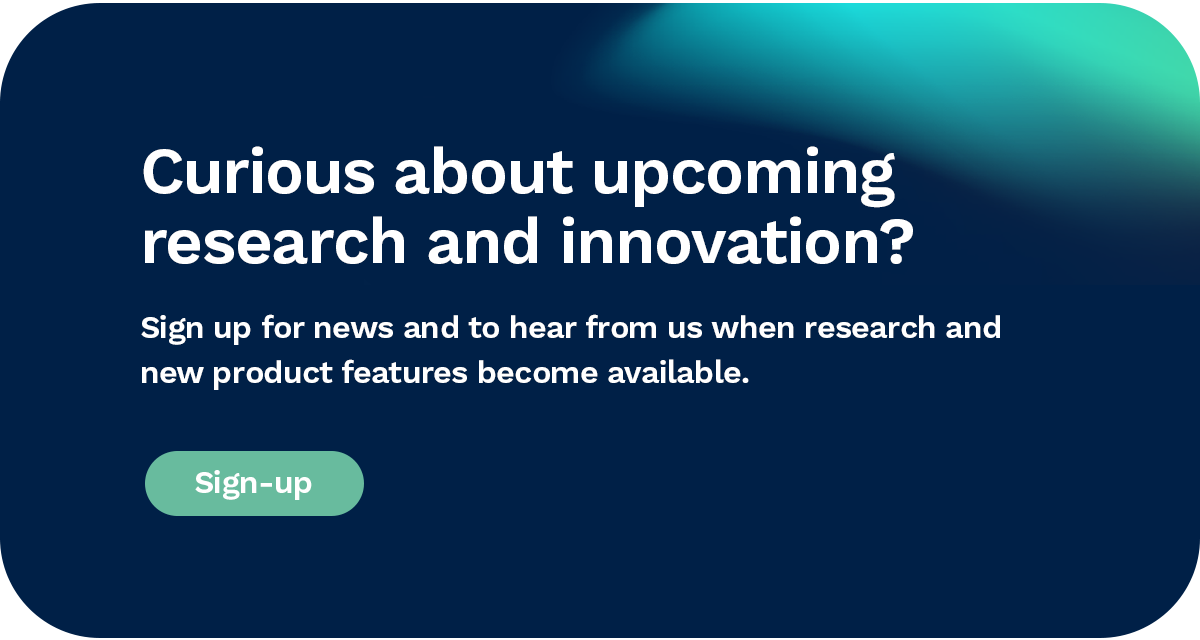