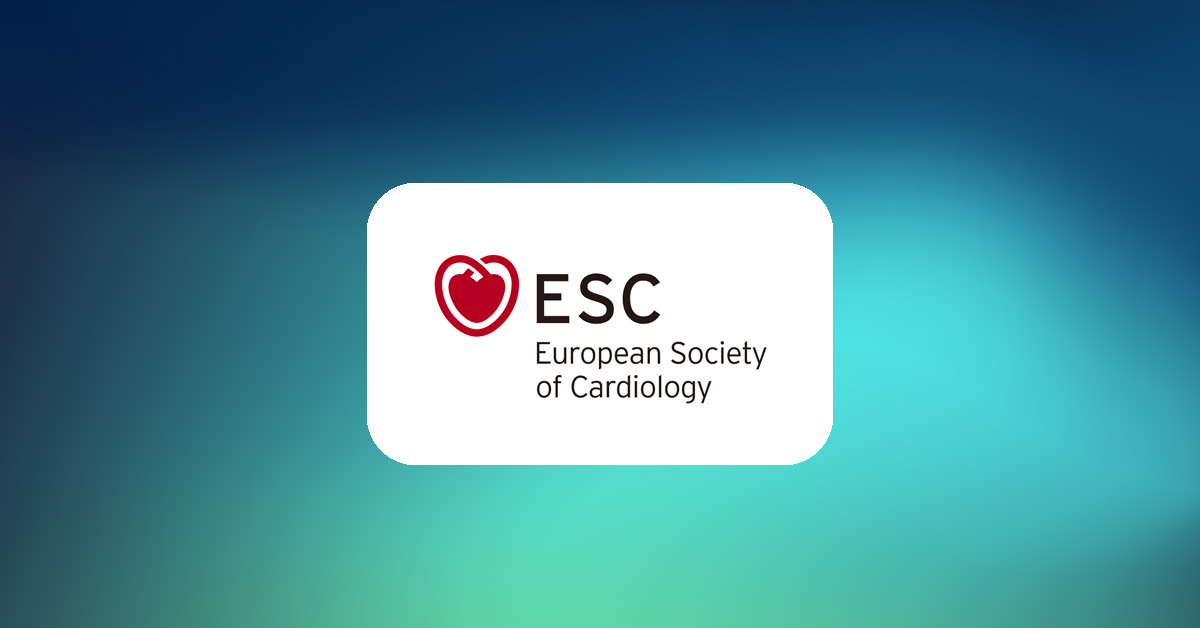
Fully Automated Left Ventricular Ejection Fraction And Global Longitudinal Strain Predicts Obstructive Coronary Artery Disease In Patients Undergoing Stress Echocardiography: A Multi-Centre Study.
- | By Ultromics
Jamie O’Driscoll, William Hawkes, Arian Beqiri, Angela Mumith, Andrew Parker, Ross Upton, Annabelle McCourt, William Woodward, Cameron Dockerill, Stephen Heitner, Mrinal Yadava, Sajiv Kaul, Rizwan Sarwar, Rajan Sharma, Gary Woodward, Paul Leeson.
Background
Stress echocardiography (SE) is one of the most widely used, accessible and cost-effective procedures for both the diagnosis and risk stratification of patients with known or suspected coronary artery disease (CAD). Quantification of the left ventricle has been shown to improve diagnostic accuracy; however, this process currently requires manual contouring and/or offline analysis. We applied an artificial intelligence (AI) pipeline capable of fully automated contouring for left ventricular ejection fraction (LVEF) and global longitudinal strain (GLS) analysis of both non-contrast and contrast SE images in a large international multi-centre population with suspected CAD.
Methods
500-patients referred for a clinically indicated SE were included (71.8% dobutamine, 28% exercise, 0.2% pacing stress). 389-patients required contrast.
All SE images were retrospectively processed using the EchoGo (Ultromics, Oxford) platform.
AI derived contours of the endocardial border were automatically generated for apical 2 and 4-chamber views. End diastolic (ED) and end systolic (ES) frame selection, and consequently cycle selection, were automatically determined by the software based upon LV volume (Figure 1).
Clinical follow-up information for up to 1 year after the SE was obtained from medical records. The principle end-point of interest was prognostically significant CAD (≥70% stenosis), defined against invasive coronary angiography.
Results
Ischaemic patients had significantly greater AI-measures of LV volumes and significantly lower LVEF and GLS (all p<0.001) at rest and peak SE.
The optimal cut-off value for stratification of CAD using automated LVEF and GLS alone (Figure 2), were 64% (sensitivity 83% and specificity 72%) and -17% (sensitivity 80% and specificity 69%) respectively.
Peak LVEF (0.93; 95% CI 0.9-0.96) and peak GLS (1.15; 95% CI 1.07-1.24) were significant independent predictors of CAD. The addition of automated LVEF and GLS to basic models significantly improved the C statistic from 0.78 to 0.83 and 0.85 (both p<0.001), respectively.
A peak LVEF <64% and peak GLS >-17% alone or in combination with the presence of myocardial ischaemia had a significantly (p<0.02) higher likelihood of identification of CAD on angiography than ischaemia alone. (Figure 3).
Conclusion
Fully automated LVEF and GLS in non-contrast and contrast SE images is feasible and independently augment the prediction of obstructive CAD above and beyond traditional SE indexes.