Andrew Parker, Arian Beqiri, Angela Mumith, Mihaela Porumb, Shan Gao, Ross Upton, Paul Leeson, Jamie O’Driscoll, Riswan Sarwar, Gary Woodward, Ultromics, Oxford, United Kingdom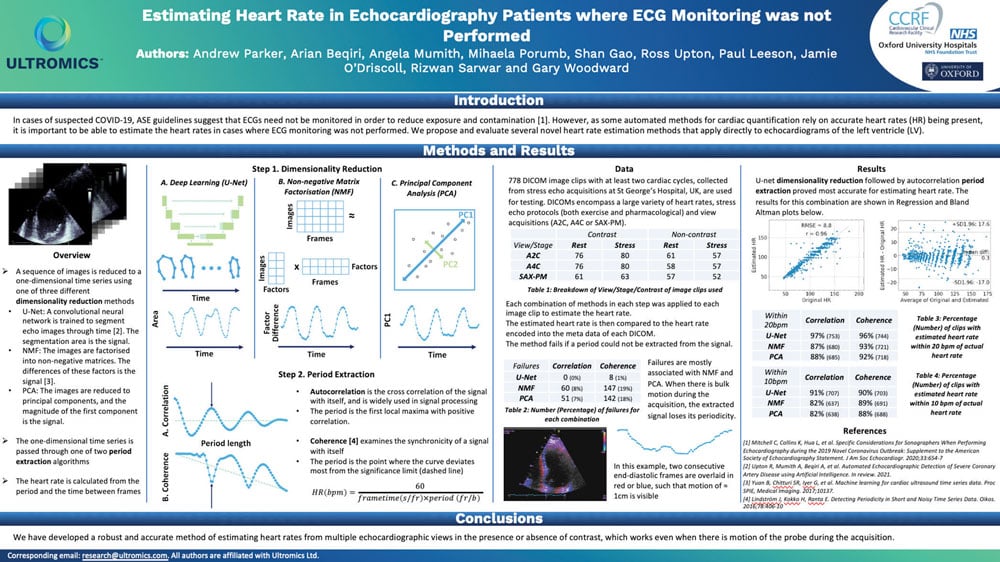
Poster of study. Click to open image in a new window.
Background
According to latest EACVI recommendations, if there is a suspected case of COVID-19, patient ECGs may not be monitored during an echocardiogram to reduce exposure and contamination. However, many cardiac parameters rely on accurate end-diastolic (ED) and end-systolic (ES) frame selection (e.g. EF and GLS), and automated methods for ED and ES extraction depend on the heart rate (HR) being present for the recorded study. Here we present a novel HR extraction method to accurately infer the heart rate from echocardiograms of the left ventricle (LV).
Methods
The HR extraction approach we take requires the reduction of image dimensionality, followed by signal period extraction. Here we attempt two dimensionality reduction methods: non-negative matrix factorisation (NNMF), and a segmentation deep-learning based method. The period was then extracted from these series using autocorrelation analysis. In total, 784 DICOM image clips from stress echo acquisitions from multiple UK sites are used for testing, where the HR encoded into the meta data is compared to the output of the method. Image clips encompass a variety of heart rates (394 are at rest, 390 are at peak), a variety of views (275 two-chamber, 275 four-chamber, 234 short axis), and with and without contrast (442 contrast, 342 non-contrast).
Results
When applying NNMF we find that the extracted signal fails to adequately factorise the image clips into ED and ES when there is any movement of the LV during acquisition, so a periodic cycle cannot be extracted in these cases. When using the automated segmentations through time, we find that accurate inference of HR is possible using auto-correlation analysis in most image clips even when motion is present. We separate our analysis by image clips taken at rest or at stress. In resting acquisitions, 87.6% (95.4%) of heart rates inferred were within 10 bpm (20 bpm) of the original HR. In stress acquisitions, 76.2% (90.5%) of heart rates were inferred within 10 bpm (20 bpm) of the original HR.
Conclusion
We have developed a robust and accurate method of inferring heart rate directly from echocardiogram images which works even in the presence of bulk motion that occurred.
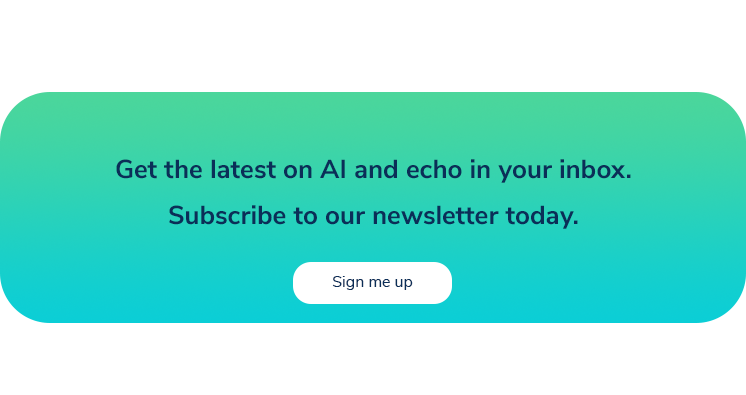