Kit M. Bransby, Woo-Jin Cho Kim, Arian Beqiri, Alex Thorley, Jorge Oliviera, Alberto Gomez and Agis Chartsias
Read the full publication here.
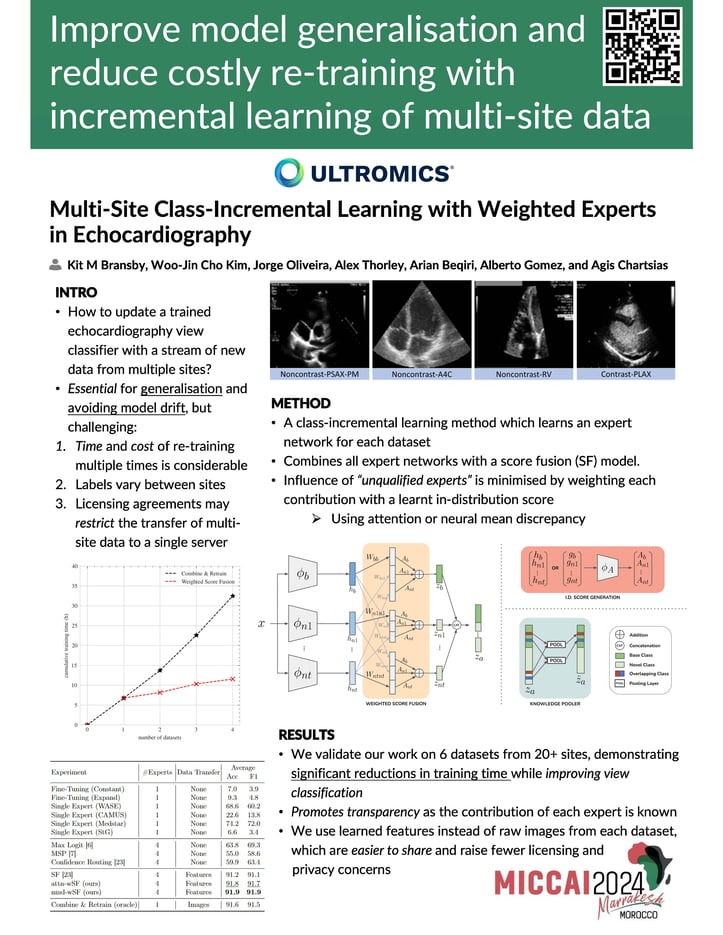
Background
Building an echocardiography view classifier that maintains performance in real-life cases requires diverse multi-site data, and frequent updates with newly available data to mitigate model drift. Simply fine-tuning on new datasets results in “catastrophic forgetting”, and cannot adapt to variations of view labels between sites. Alternatively, collecting all data on a single server and re-training may not be feasible as data sharing agreements may restrict image transfer, or datasets may only become available at different times. Furthermore, time and cost associated with re-training grows with every new dataset. We propose a class-incremental learning method which learns an expert network for each dataset, and combines all expert networks with a score fusion model. The influence of “unqualified experts” is minimised by weighting each contribution with a learnt in-distribution score. These weights promote transparency as the contribution of each expert is known during inference. Instead of using the original images, we use learned features from each dataset, which are easier to share and raise fewer licensing and privacy concerns. We validate our work on six datasets from multiple sites, demonstrating significant reductions in training time while improving view classification performance.
Read the full publication here.
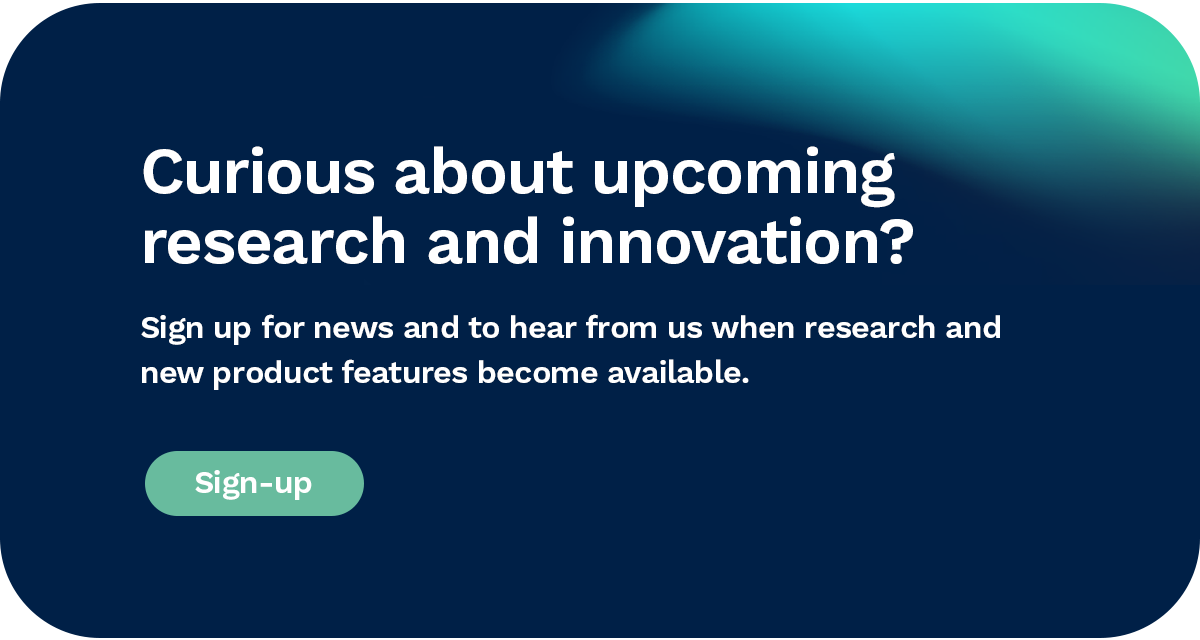